Fact-Checking through Crowd-sourcing Generative Models
Traditional fact-checking methods are crucial but often expensive and difficult to scale. Could there be a more efficient way? This study explores a groundbreaking approach that leverages large language models to create "silicon subjects" for fact-checking, offering a scalable and cost-effective solution to combat misinformation.
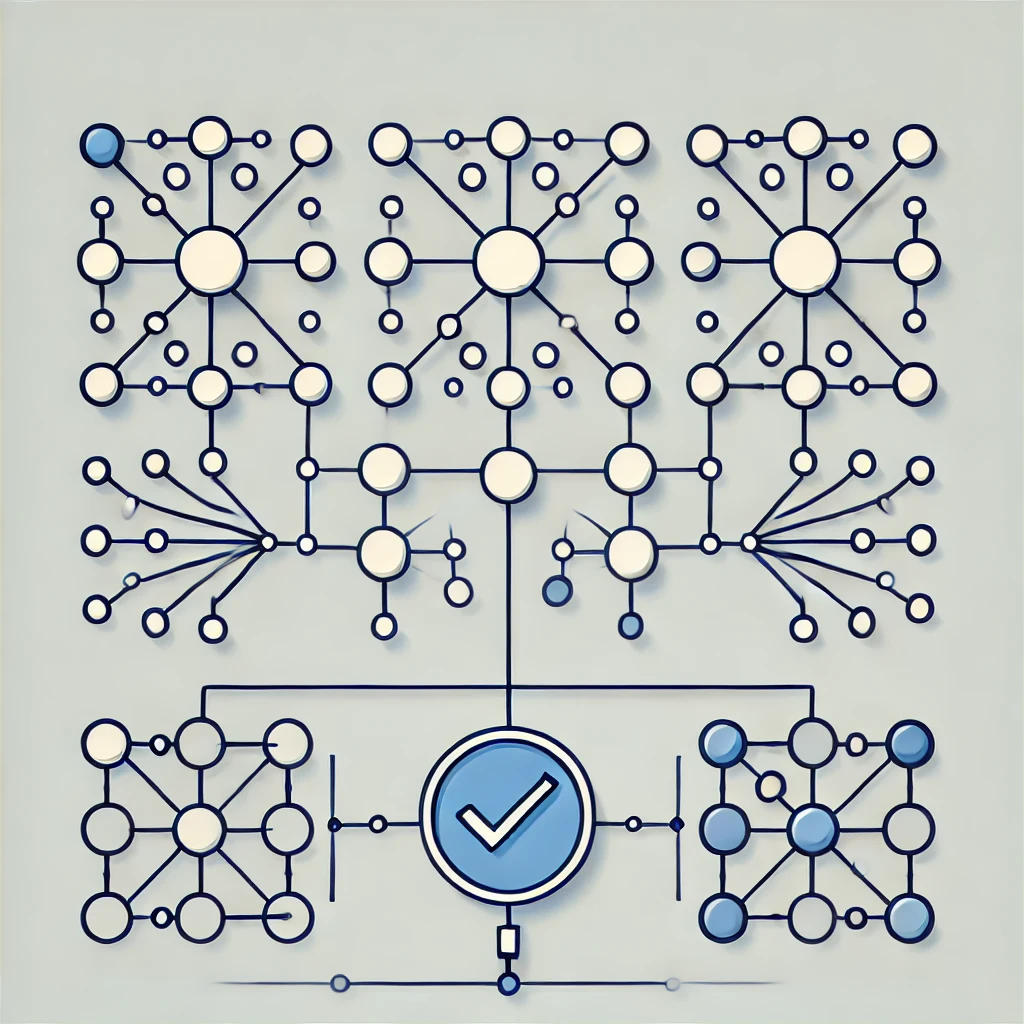
In the digital age, the proliferation and rapid dissemination of information, particularly via social media, have made it easy for false information to spread more swiftly than verified facts, resulting in profound social, political, economic, and environmental repercussions. Traditional fact-checking methods, while essential, are often costly and challenging to scale. Crowdsourcing presents an alternative but is hindered by individual biases. This study investigates an innovative approach proposed by Ding and Johar (2022), which suggests utilizing similarity judgments over direct veracity judgments to mitigate bias. Building on this, we explore the use of large language models (LLMs) to generate silicon subjects for performing similarity judgments. Using GPT-4o, we created 521 silicon subjects to rate the similarity of fifty climate change articles, replicating Ding and Johar's methodology. Our results demonstrate that silicon subjects can achieve accuracy comparable to, and occasionally surpassing, human subjects in similarity assessments based on expert-rated veracity scores. This research highlights the potential of LLMs in enhancing fact-checking processes, providing a scalable and cost-effective alternative to traditional methods.